各不相同的 5类的图形,每类100张
import numpy as npfrom keras.datasets import mnistimport gcfrom keras.models import Sequential, Modelfrom keras.layers import Input, Dense, Dropout, Flattenfrom keras.layers.convolutional import Conv2D, MaxPooling2Dfrom keras.applications.vgg16 import VGG16from keras.optimizers import SGDfrom keras.utils.data_utils import get_fileimport cv2import h5py as h5py import numpy as npimport osimport mathfrom matplotlib import pyplot as plt#全局变量RATIO = 0.2train_dir = 'D:/dl4cv/datesets/littleCBIR/'#根据分类总数确定one-hot总类NUM_DENSE = 5#训练总数epochs = 10def tran_y(y): y_ohe = np.zeros(NUM_DENSE) y_ohe[y] = 1 return y_ohe#根据Ratio获得训练和测试数据集的图片地址和标签##生成数据集,本例先验3**汽车、4**恐龙、5**大象、6**花、7**马def get_files(file_dir, ratio): ''' Args: file_dir: file directory Returns: list of images and labels ''' image_list = [] label_list = [] for file in os.listdir(file_dir): if file[0:1]=='3': image_list.append(file_dir + file) label_list.append(0) elif file[0:1]=='4': image_list.append(file_dir + file) label_list.append(1) elif file[0:1]=='5': image_list.append(file_dir + file) label_list.append(2) elif file[0:1]=='6': image_list.append(file_dir + file) label_list.append(3) else: image_list.append(file_dir + file) label_list.append(4) print('数据集导入完毕') #图片list和标签list #hstack 水平(按列顺序)把数组给堆叠起来 image_list = np.hstack(image_list) label_list = np.hstack(label_list) temp = np.array([image_list, label_list]) temp = temp.transpose() np.random.shuffle(temp) all_image_list = temp[:, 0] all_label_list = temp[:, 1] n_sample = len(all_label_list) #根据比率,确定训练和测试数量 n_val = math.ceil(n_sample*ratio) # number of validation samples n_train = n_sample - n_val # number of trainning samples tra_images = [] val_images = [] #按照0-n_train为tra_images,后面位val_images的方式来排序 for index in range(n_train): image = cv2.imread(all_image_list[index]) #灰度,然后缩放 image = cv2.cvtColor(image,cv2.COLOR_RGB2GRAY) image = cv2.resize(image,(48,48))#到底在这个地方修改,还是在后面修改,需要做具体实验 tra_images.append(image) tra_labels = all_label_list[:n_train] tra_labels = [int(float(i)) for i in tra_labels] for index in range(n_val): image = cv2.imread(all_image_list[n_train+index]) #灰度,然后缩放 image = cv2.cvtColor(image,cv2.COLOR_RGB2GRAY) image = cv2.resize(image,(32,32)) val_images.append(image) val_labels = all_label_list[n_train:] val_labels = [int(float(i)) for i in val_labels] return np.array(tra_images),np.array(tra_labels),np.array(val_images),np.array(val_labels)# colab+VGG要求至少48像素在现有数据集上,已经能够完成不错情况ishape=48#(X_train, y_train), (X_test, y_test) = mnist.load_data() #获得数据集#X_train, y_train, X_test, y_test = get_files(train_dir, RATIO)#保持数据##np.savez("D:\\dl4cv\\datesets\\littleCBIR.npz",X_train=X_train,y_train=y_train,X_test=X_test,y_test=y_test)#读取数据 path='littleCBIR.npz'#https://github.com/jsxyhelu/GOCW/raw/master/littleCBIR.npzpath = get_file(path,origin='https://github.com/jsxyhelu/GOCW/raw/master/littleCBIR.npz')f = np.load(path)X_train, y_train = f['X_train'], f['y_train']X_test, y_test = f['X_test'], f['y_test']X_train = [cv2.cvtColor(cv2.resize(i, (ishape, ishape)), cv2.COLOR_GRAY2BGR) for i in X_train] X_train = np.concatenate([arr[np.newaxis] for arr in X_train]).astype('float32') X_train /= 255.0X_test = [cv2.cvtColor(cv2.resize(i, (ishape, ishape)), cv2.COLOR_GRAY2BGR) for i in X_test] X_test = np.concatenate([arr[np.newaxis] for arr in X_test]).astype('float32')X_test /= 255.0y_train_ohe = np.array([tran_y(y_train[i]) for i in range(len(y_train))]) y_test_ohe = np.array([tran_y(y_test[i]) for i in range(len(y_test))])y_train_ohe = y_train_ohe.astype('float32')y_test_ohe = y_test_ohe.astype('float32')model_vgg = VGG16(include_top = False, weights = 'imagenet', input_shape = (ishape, ishape, 3)) #for i, layer in enumerate(model_vgg.layers): # if i<20:for layer in model_vgg.layers: layer.trainable = Falsemodel = Flatten()(model_vgg.output) model = Dense(4096, activation='relu', name='fc1')(model)model = Dense(4096, activation='relu', name='fc2')(model)model = Dropout(0.5)(model)model = Dense(NUM_DENSE, activation = 'softmax', name='prediction')(model) model_vgg_pretrain = Model(model_vgg.input, model, name = 'vgg16_pretrain')#model_vgg_pretrain.summary()print("vgg准备完毕\n")sgd = SGD(lr = 0.05, decay = 1e-5) model_vgg_pretrain.compile(loss = 'categorical_crossentropy', optimizer = sgd, metrics = ['accuracy'])print("vgg开始训练\n")log = model_vgg_pretrain.fit(X_train, y_train_ohe, validation_data = (X_test, y_test_ohe), epochs = epochs, batch_size = 64)score = model_vgg_pretrain.evaluate(X_test, y_test_ohe, verbose=0)print('Test loss:', score[0])print('Test accuracy:', score[1])plt.figure('acc') plt.subplot(2, 1, 1) plt.plot(log.history['acc'],'r--',label='Training Accuracy') plt.plot(log.history['val_acc'],'r-',label='Validation Accuracy') plt.legend(loc='best') plt.xlabel('Epochs') plt.axis([0, epochs, 0.5, 1]) plt.figure('loss') plt.subplot(2, 1, 2) plt.plot(log.history['loss'],'b--',label='Training Loss') plt.plot(log.history['val_loss'],'b-',label='Validation Loss') plt.legend(loc='best') plt.xlabel('Epochs') plt.axis([0, epochs, 0, 1]) plt.show() os.system("pause")
epoch = 10
应该说,主要代码问题不大,也摸索出来了系列方法,但是需要进一步丰富。今天,我在之前的基础上继续研究,解决以下问题:
1、在 5Type4CBIR( https://www.kaggle.com/jsxyhelu/5type4cbir) 做训练;
2、使用aumentation方法,提高前期效果;
3、 不仅仅是曲线, 结果要以可视化方法绘制出来;
4、最终的结果要能够下载下来;
1、在 5Type4CBIR( https://www.kaggle.com/jsxyhelu/5type4cbir) 做训练 ;
基于之前的积累,我已经将数据集变成了npz格式,可以直接使用keras进行下载。为此,我专(peng)门(qiao)在kaggle上创建了这个数据集,立刻感到B格高涨。

kaggle上传太慢,npz的数据仍然从github中下载;
2、使用aumentation方法,提高前期效果;
如果从一般意义上来说,aumentation方法的引入肯定能够提高处理效果的,特别对于这样一个数据集比较小的问题来说尤其是这样。从目前这个意义上来看:
img_generator.fit(X_train)
# fits the model_2 on batches with real-time data augmentation:
log = model_vgg_pretrain.fit_generator(img_generator.flow(X_train,y_train_ohe, batch_size= 64),
steps_per_epoch= len(X_train), epochs=epochs)
score = model_vgg_pretrain.evaluate(X_test, y_test_ohe, verbose= 0)
简单几行代码,就可以解决这里的问题。如果确实有效,那么将被复用;
在不generate下,达到95
其中,核心代码及参数为:
log = model_vgg_pretrain.fit_generator(img_generator.flow(X_train,y_train_ohe, batch_size= 128), steps_per_epoch = 400, epochs=10,validation_data=(X_test, y_test_ohe),workers=4)
反观数据,可以发现在epoch = 7 的时候,就已经达到最好;这个曲线较前面的训练更为平滑,总体质量也更高。98的准确率对于消费级的应用是一个很好的结果。
augumentation的方法将会被经常复用。
3、不仅仅是曲线,结果要以可视化方法绘制出来;
这里的意思也很直接,就是我要真实地去验证数据,要把模型结果显示出来,而不仅仅是在模型里面fit。这个显示首先是在juypter里面直观的观看。这个是给我来看的。一个是要在后面的结果应用中能够下载,并且被我现在的软件来使用。
但是目前这一步无法完成,因为前期我使用OpenCV读入图像、预处理、压制成npz格式的,目前我认为这个效果很好。OpenCV的 Mat格式目前无法在Juypter中进行显示。
4、最终的结果要能够下载下来;
colab中有一段可以下载的代码的,翻阅了一些资料,解决了这个问题:
# Install the PyDrive wrapper & import libraries.# This only needs to be done once in a notebook.!pip install -U -q PyDrivefrom pydrive.auth import GoogleAuthfrom pydrive.drive import GoogleDrivefrom google.colab import authfrom oauth2client.client import GoogleCredentials# Authenticate and create the PyDrive client.# This only needs to be done once in a notebook.auth.authenticate_user()gauth = GoogleAuth()gauth.credentials = GoogleCredentials.get_application_default()drive = GoogleDrive(gauth)# Create & upload a text file.uploaded = drive.CreateFile()uploaded.SetContentFile('5type4cbirMODEL.h5')uploaded.Upload()print('Uploaded file with ID {}'.format(uploaded.get('id')))
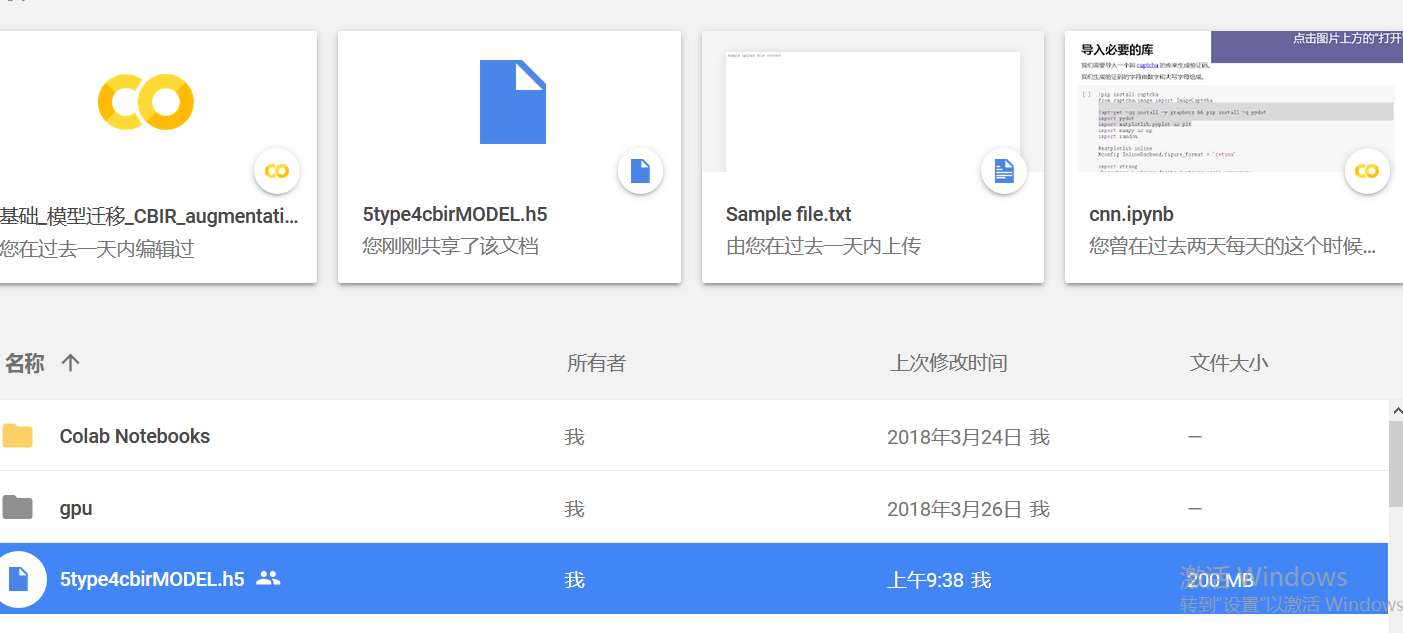
但是这样一个200m的东西下载下来也比较费时间,如果能够结合google driver直接使用是最好的。google driver就相当于colab的外存储。调用的代码应该类似这样:
# Install the PyDrive wrapper & import libraries.# This only needs to be done once per notebook.!pip install -U -q PyDrivefrom pydrive.auth import GoogleAuthfrom pydrive.drive import GoogleDrivefrom google.colab import authfrom oauth2client.client import GoogleCredentials# Authenticate and create the PyDrive client.# This only needs to be done once per notebook.auth.authenticate_user()gauth = GoogleAuth()gauth.credentials = GoogleCredentials.get_application_default()drive = GoogleDrive(gauth)#根据文件名进行下载file_id = '1qjxAm_QiXdSqBmyIoPl3bfnyLNJxwKo9'downloaded = drive.CreateFile({ 'id': file_id})print('Downloaded content "{}"'.format(downloaded.GetContentString()))
还是要通过id来进行调用。毕竟这个调用只会发生在内部情况下,可以理解。
到了小结时间了 :
昨天和今天在做CBIR的实验,得到目前这个结果,应该说能够解决一些问题,特别是模型训练这个方面的问题了,还是有很多收获的,当然主要的收获还是在代码里面:
1、agumenntation可以提高质量,fit_generate也是有技巧的(step_per_epoch),更不要说在数据集准备这块前期积累的技巧;
2、transform是可以用来解决垂直领域问题的,下一步就是需要验证;
3、研究问题的时候,首先是不能失去信心,然后就是要总结方法。
附件列表